Table of Contents Hide
Understanding the 5 Characteristics of Data for Success
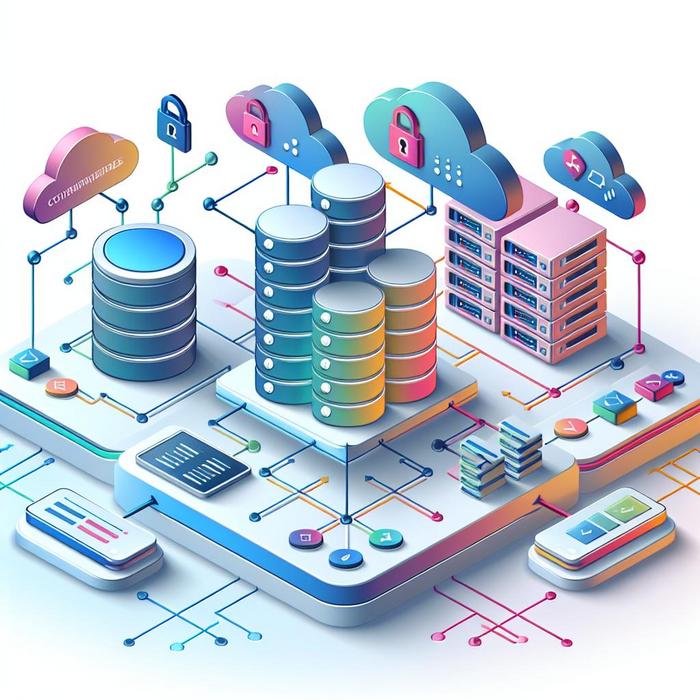
Data Accuracy: The Cornerstone Of Reliable Insights
Is your organization struggling to derive meaningful insights from its data? In today’s data-driven landscape, the accuracy of information is paramount. Decisions based on flawed data can lead to costly mistakes and missed opportunities. Ensuring data accuracy requires a multi-faceted approach, encompassing data validation at entry points, consistent data formatting, and ongoing data quality monitoring. This commitment to accurate data lays the foundation for informed decision-making and empowers organizations to gain a competitive edge. By prioritizing data accuracy from the outset, businesses can establish a strong base for all subsequent data operations. This proactive approach minimizes the risk of errors propagating through the system and ensures the reliability of insights derived from the data.
Data Completeness: Filling The Gaps For A Holistic View
How can you ensure your data provides a complete picture of your business operations? Incomplete data sets hinder comprehensive analysis and can skew insights, leading to inaccurate conclusions. Data completeness focuses on capturing all necessary information across various data sources and minimizing missing values. Implementing strategies for data completeness, such as mandatory fields in data entry forms and regular data audits, ensures that your data provides a holistic view of your organization’s performance. Addressing data completeness early on, during the data collection and integration phases, prevents downstream issues in analysis and reporting. This proactive approach saves valuable time and resources that would otherwise be spent on rectifying data gaps later in the process.
Data Consistency: Harmonizing Information Across Platforms
What challenges do inconsistencies across different data platforms pose to your organization? Data consistency is crucial for maintaining data integrity and enabling seamless integration across multiple systems. Inconsistent data can lead to conflicting reports, inaccurate analyses, and ultimately, flawed business decisions. Maintaining data consistency involves implementing data governance policies, standardizing data formats, and employing database virtualization techniques to create a unified view of data, regardless of its source. For instance, consider a scenario where sales data is stored in one system and marketing data in another. Without consistency, it becomes challenging to analyze the effectiveness of marketing campaigns on sales performance accurately. Database virtualization can streamline access to this information, presenting a consistent, unified view for analysis. This approach enhances the efficiency of data utilization and facilitates informed decision-making. You can find more information on data quality here.
Data Timeliness: Accessing Information When It Matter Most
How quickly can your organization access the data it needs to make critical decisions? Data timeliness refers to the availability of data when it’s needed for analysis and decision-making. In today’s fast-paced business environment, outdated information can quickly become irrelevant. Implementing real-time data ingestion and processing pipelines ensures that decision-makers have access to the most current information, enabling them to respond quickly to changing market dynamics. By ensuring access to fresh, relevant data, businesses can make agile decisions, capitalize on opportunities, and mitigate risks effectively. Cloud solutions can play a pivotal role in achieving data timeliness by providing scalable and efficient data processing capabilities. Furthermore, consider a scenario where a financial institution needs to assess a customer’s creditworthiness in real-time for loan approval. Accessing up-to-the-minute financial data is crucial for making an accurate and timely decision. This emphasizes the critical role of data timeliness in various business contexts.
Data Validity: Ensuring Data Confirms To Defined Standards
Are you confident that your data adheres to the required standards and regulations? Data validity ensures that data conforms to predefined rules and formats, guaranteeing its reliability and usability for analysis. Implementing data validation checks at various stages of the data lifecycle prevents errors and ensures that data meets the necessary quality standards. This includes verifying data types, enforcing data constraints, and performing data anonymization to protect sensitive information while maintaining data utility. For example, in healthcare, patient data must adhere to strict privacy regulations. Data anonymization techniques allow researchers and analysts to access valuable information while safeguarding patient confidentiality. Automating compliance using software tools can streamline these processes. Ensuring compliance with these standards is not only crucial for ethical considerations but also for maintaining the organization’s reputation and avoiding potential legal repercussions. You can explore further insights into the characteristics of effective data science practices here.
Compering Data Management Platforms: A Strategic Imperative
How do you navigate the complex landscape of data management platforms and select the right solution for your organization’s unique needs? Choosing the appropriate data management platform is a crucial decision for any organization. Different platforms offer varying capabilities in terms of data integration, data quality management, data governance, and data security. A thorough comparison of available platforms, considering factors like scalability, performance, cost, and compliance features, is essential for making an informed decision. This comparative analysis should align with the organization’s specific data strategy and long-term goals. The selection process involves assessing the platform’s ability to handle the organization’s data volume and velocity, its integration capabilities with existing systems, and its adherence to relevant industry regulations. A well-informed decision in this area contributes significantly to the organization’s overall data management effectiveness. The implications of data quality on healthcare research are discussed further here.
Ensuring Data Security And Compliance
How do you effectively balance data accessibility with robust security and compliance requirements? In today’s regulatory landscape, safeguarding sensitive data is paramount. Data security measures, including access controls, encryption, and data anonymization techniques, are crucial for protecting data from unauthorized access and breaches. Moreover, organizations must comply with various data privacy regulations, such as GDPR and CCPA. Implementing robust data governance frameworks, conducting regular security audits, and leveraging data anonymization tools help organizations maintain compliance and build trust with their stakeholders. This proactive approach to data security and compliance not only protects sensitive information but also safeguards the organization’s reputation and mitigates potential legal risks. By prioritizing these aspects, organizations demonstrate their commitment to responsible data handling and foster a culture of data integrity. Choosing the right security testing tools is also essential for maintaining robust data security.
Implementing Effective Data Govermence
What strategies can organizations employ to establish and maintain effective data governance practices? Data governance provides the framework for managing data assets throughout their lifecycle. Implementing clear data policies, defining data ownership and responsibilities, and establishing data quality standards are essential components of a robust data governance program. These practices ensure data consistency, accuracy, and compliance with regulatory requirements. Effective data governance also facilitates collaboration between different departments and stakeholders, fostering a shared understanding of data management principles. By implementing these strategies, organizations can optimize data utilization, improve decision-making, and minimize risks associated with data mismanagement. This proactive approach to data governance establishes a foundation for responsible and effective data management across the organization.
Streamlining Data Provisioning For Agility
How can organizations accelerate the delivery of data to their development and testing environments? Efficient data provisioning is essential for supporting agile development methodologies and accelerating the software development lifecycle. Traditional data provisioning methods can be slow and cumbersome, hindering the speed of innovation. Implementing database virtualization allows organizations to create lightweight, on-demand copies of production data for development and testing purposes, significantly reducing provisioning time and infrastructure costs. This approach empowers development teams to access realistic data quickly, facilitating rapid prototyping, continuous integration, and faster time-to-market for new applications and features. By streamlining data provisioning processes, organizations can gain a competitive edge in today’s dynamic market. Optimizing realistic data for mobile applications is a crucial aspect of modern software development.
Ensuring Data Privacy With Anonymization Techniques
How can organizations protect sensitive data while still leveraging its value for development and testing? Data anonymization plays a vital role in safeguarding sensitive information while maintaining data utility for non-production environments. Techniques like data masking, pseudonymization, and tokenization allow organizations to replace sensitive data elements with realistic but non-sensitive substitutes. This ensures that development and testing teams can work with data that mimics real-world scenarios without compromising the privacy of individuals. By implementing effective data anonymization techniques, organizations can comply with data privacy regulations and build trust with their customers. Decoding data anonymization techniques provides a deeper understanding of this crucial process. You can learn more about the characteristics of high-quality data here.
Oopimizing Data Subsetting For Efficiency
How can organizations reduce storage costs and improve performance by working with smaller, focused data sets? Data subsetting involves extracting a smaller, representative portion of a larger data set for specific purposes, such as development, testing, or analytics. By working with smaller data sets, organizations can reduce storage costs, improve processing speed, and simplify data management tasks. Effective data subsetting techniques ensure that the subset accurately reflects the characteristics of the original data, preserving its statistical properties and ensuring reliable results. This targeted approach to data utilization optimizes resource allocation and enables more efficient data analysis and processing.
Leveraging Data Virtualization For Cost Savings
What advantages does database virtualization offer in terms of cost reduction and resource optimization? Database virtualization provides a cost-effective alternative to traditional data provisioning methods. By creating virtual copies of databases, organizations can reduce storage requirements, minimize hardware costs, and streamline data management processes. This approach eliminates the need for multiple physical copies of data, freeing up valuable IT resources and reducing operational expenses. Database virtualization also enhances data accessibility and accelerates development cycles, contributing to overall cost savings and improved efficiency. You can explore more about characteristics of data here.
Implementing Robust Data Validation Procedures
How can organizations ensure the integrity and reliability of their data through robust validation procedures? Data validation involves verifying that data conforms to predefined rules, formats, and constraints. Implementing automated data validation checks throughout the data lifecycle helps identify and correct errors early on, preventing the propagation of inaccurate information. These checks ensure data quality, maintain data integrity, and support reliable decision-making. Robust data validation procedures are essential for building trust in data and ensuring its fitness for purpose. You can find data sets for testing and analysis here.
Maintaining Data Integrity Across Multiple Systems
What strategies can be employed to ensure data remains consistent and accurate across diverse systems and platforms? Maintaining data integrity across multiple systems is a critical challenge for organizations. Implementing data synchronization mechanisms ensures that data remains consistent across various platforms, eliminating data silos and enabling a unified view of information. This approach supports accurate reporting, informed decision-making, and seamless data integration across the organization. By prioritizing data integrity, organizations can optimize data utilization and foster a culture of data-driven insights. Mastering data privacy regulations is essential in this context.
Choosing The Right Data Platform For Your Business
What key considerations should guide organizations in selecting the optimal data management platform for their specific needs? Selecting the right data management platform is a strategic decision that should align with an organization’s business objectives and data strategy. Factors to consider include scalability, performance, security, integration capabilities, and cost. Evaluating different platform options based on these criteria helps organizations identify the solution that best meets their unique requirements and supports their long-term data management goals. A well-chosen data platform empowers organizations to effectively manage their data assets and unlock the full potential of their data. Critical characteristics of data-driven organizations are further explored here.