Table of Contents Hide
Elevating Data Operations with Scalable Test Data Management
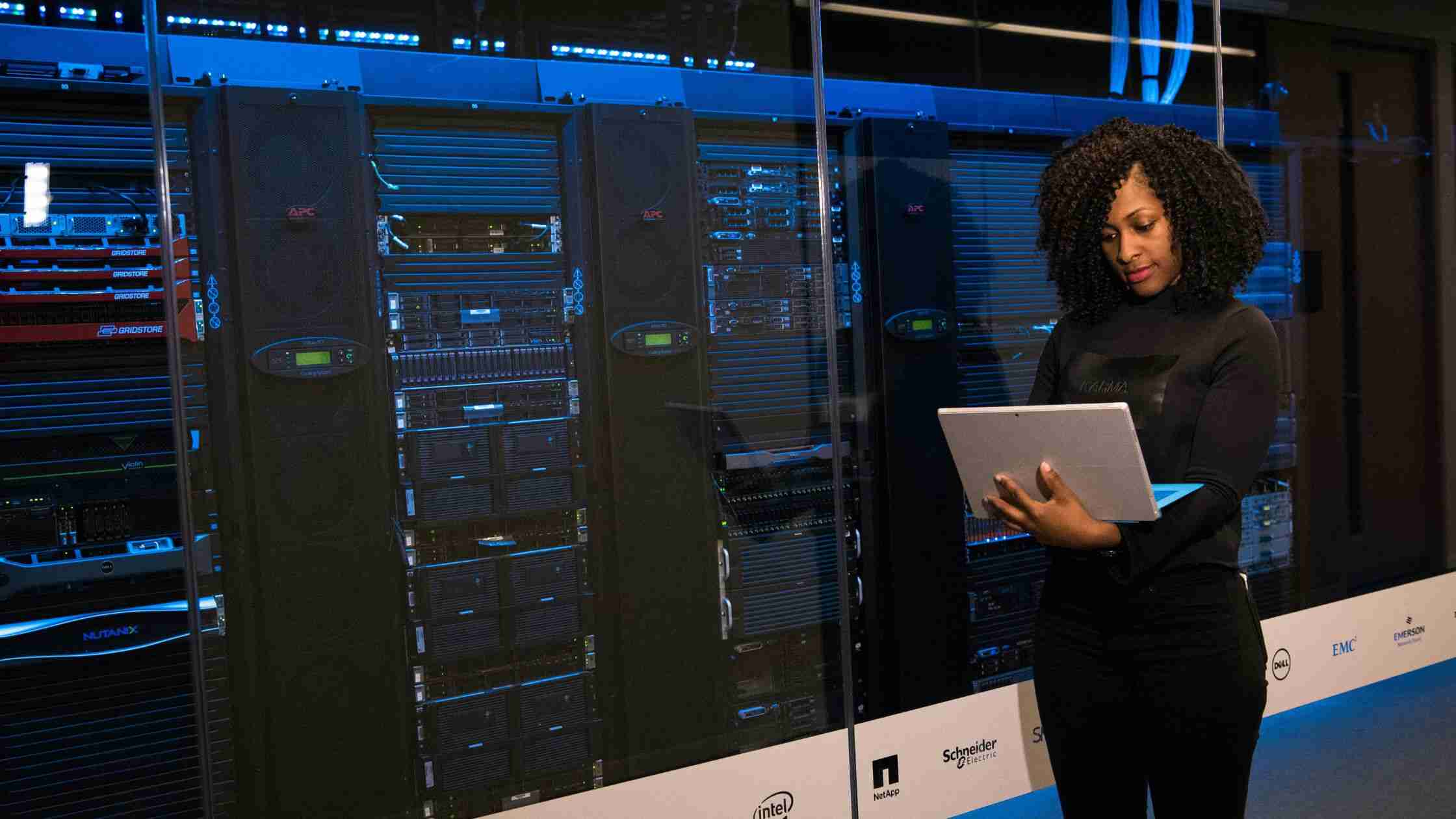
What is DataOps?
In today’s fast-paced digital landscape, businesses are inundated with vast amounts of data. Managing, analyzing, and utilizing this data efficiently is a critical challenge. Enter DataOps—a methodology that transforms how organizations approach data operations, ensuring speed, accuracy, and scalability. But what is DataOps? More than just a buzzword, data operations is a comprehensive framework that aligns data management practices with operational processes, bridging the gap between data engineers, data scientists, and IT operations. This blog delves into the core concepts of data operations, its importance in modern enterprises, and how Accelario’s tools can enhance your DataOps strategy.
A Modern Approach to Data Operations
DataOps, short for data operations, is a set of practices, processes, and technologies aimed at improving the efficiency and effectiveness of data analytics. It borrows principles from DevOps, focusing on collaboration, automation, and continuous improvement. Unlike traditional data management approaches, data operations emphasizes agility, aiming to deliver data faster while maintaining high quality.
The Rise
As data-driven decision-making becomes the norm, the traditional data pipeline—characterized by slow, linear processes—fails to keep pace with the demands of modern businesses. DataOps emerged to address this gap, promoting a more agile and automated approach to data management. This shift is especially crucial as organizations increasingly rely on real-time data for strategic decisions.
Key Components of a DataOps Framework
A successful data operations framework is built on several key components:
- Collaboration and Communication: Data operations fosters a culture of collaboration between data engineers, data scientists, and IT operations teams. This cross-functional cooperation ensures that data flows seamlessly from collection to analysis.
- Automation: By automating repetitive tasks, DataOps reduces human error and accelerates data processing. Automation tools can handle everything from data ingestion to quality checks, freeing up teams to focus on more strategic tasks.
- Monitoring and Measurement: Continuous monitoring of data processes allows teams to detect and rectify issues in real-time. Data operations emphasizes metrics and KPIs to ensure that data operations are aligned with business goals.
- Continuous Integration and Delivery (CI/CD): Borrowing from DevOps, DataOps applies CI/CD practices to data management, enabling rapid updates to data pipelines and analytics tools.
- AI Integration: The rise of AI DataOps adds a layer of intelligence to the framework, leveraging machine learning algorithms to optimize data operations. AI-driven analytics can predict trends, detect anomalies, and suggest improvements to the data pipeline.
Benefits of Implementing Data Operations
Adopting a data operations framework offers numerous benefits, including:
- Faster Time-to-Insight: Data operations accelerates the delivery of data insights, enabling businesses to make decisions based on the most current information.
- Improved Data Quality: With automated testing and monitoring, DataOps ensures that data is accurate and reliable, reducing the risk of errors.
- Scalability: As organizations grow, so does their data. Data operations provides a scalable framework that can handle increasing data volumes without compromising performance.
- Enhanced Collaboration: By breaking down silos between teams, DataOps fosters a collaborative environment where everyone works towards a common goal.
Integrating Test Data Management with DataOps
As the DataOps framework gains traction in modern enterprises, the role of Test Data Management (TDM) becomes increasingly significant. TDM ensures that test environments are populated with accurate, secure, and high-quality data—crucial for validating the integrity of data operations. Integrating TDM with DataOps not only streamlines the testing process but also enhances the overall efficiency and reliability of data pipelines.
The Role of Test Data Management in DataOps
In a DataOps framework, the continuous flow of data across various stages of the pipeline requires rigorous testing to ensure quality and compliance. Here’s how TDM fits into the DataOps process:
- Automated Test Data Provisioning: In a fast-paced DataOps environment, manual provisioning of test data can lead to bottlenecks. Automated TDM tools, like those offered by Accelario, can instantly generate and provide relevant test data for each stage of the pipeline, ensuring that testing keeps pace with data processing.
- Data Quality Assurance: Test Data Management helps maintain data quality by ensuring that the data used for testing mirrors production data as closely as possible. This is essential in a data operations framework, where the accuracy of test data directly impacts the reliability of data operations.
- Data Anonymization for Compliance: Compliance with data protection regulations, such as GDPR, is a critical concern in DataOps. TDM tools that include data anonymization features ensure that sensitive data is protected during testing, aligning with the security standards required in a DataOps environment.
- Environment Consistency: TDM ensures that test environments are consistent and reflective of production environments, reducing the risk of issues when changes are deployed. In a DataOps framework, this consistency is vital for achieving seamless integration and continuous delivery.
How Accelario Supports Test Data Management for DataOps Teams
Accelario’s suite of tools is designed to align perfectly with the principles of data operations, offering robust solutions that streamline data operations and drive efficiency.
Database Virtualization for Agile DataOps
One of the critical challenges in DataOps is managing multiple environments for development, testing, and production. Accelario’s Database Virtualization solution allows teams to create virtual copies of databases quickly, reducing the time and resources required to provision environments. This agility is essential for a DataOps framework that demands rapid iteration and continuous delivery.
Data Anonymization for Compliance and Security
Data security and compliance are top concerns in any DataOps strategy. Accelario’s Data Anonymization features ensure that sensitive information is protected throughout the data pipeline. By automating the anonymization process, Accelario helps organizations comply with regulations like GDPR while maintaining the integrity of their data.
Seamless Integration with Existing Tools
Accelario’s platform integrates seamlessly with a wide range of data management and analytics tools, making it easier for organizations to adopt data operations without overhauling their existing infrastructure. This flexibility is crucial for businesses looking to implement DataOps gradually and without disruption.
Best Practices for Implementing DataOps
When implementing DataOps, it’s essential to follow best practices to maximize its benefits:
- Start Small: Begin with a pilot project to test and refine your DataOps framework before scaling.
- Invest in Automation: Identify repetitive tasks that can be automated to free up resources for higher-value work.
- Foster Collaboration: Encourage cross-functional teams to work together, sharing knowledge and best practices.
- Focus on Continuous Improvement: Regularly review and refine your data operations processes to ensure they continue to meet business needs.
- Leverage Tools like Accelario: Use specialized tools to enhance your DataOps framework, improving efficiency and scalability.
Conclusion
DataOps is more than just a trend; it’s a transformative approach to managing data in the modern enterprise. By adopting a data operations framework, organizations can accelerate their data workflows, improve collaboration, and deliver high-quality insights faster than ever before. With tools like Accelario, implementing data operations becomes a seamless process, empowering your team to focus on what matters most—turning data into actionable insights.