Table of Contents Hide
How Data Masking Can Protect Your Healthcare Data
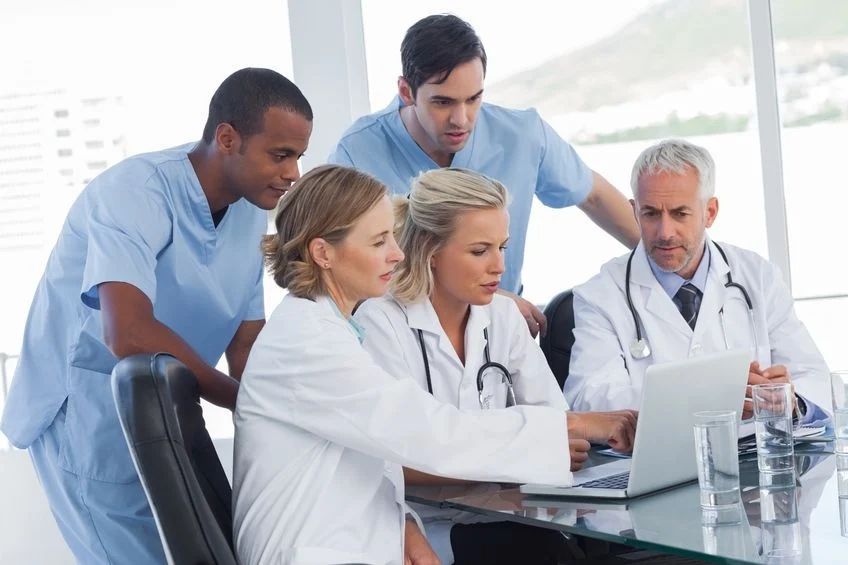
Essentially, data masking creates a duplicate of a database and replaces sensitive data with fictitious data that preserves the data’s structure and format. For instance, a financial institution may use data masking to safeguard its customers’ social security numbers or account numbers while enabling the testing and development of new applications with a production-like dataset. Credit card numbers, social security numbers, and names can all be masked to protect sensitive information without compromising the data set’s integrity.
Data masking is crucial when third-party vendors or offshore teams are handling sensitive data. Companies can use it to ensure regulatory compliance without denying developers, analysts, and testers the ability to work with real-time datasets.
We all know by now that data privacy and security are necessary to protect sensitive information from unauthorized access, theft, or misuse. The consequences of failing to protect data can be severe; it could easily result in financial loss, legal liability, reputational damage, and loss of customer trust.
How to Prevent Security Breaches in Healthcare
The ability of data masking to reduce the risk of data breaches and theft by limiting access to sensitive information is arguably what makes it most useful. Even if an attacker gains unauthorized access to a masked data set, the information is useless without the means to de-identify the data.
Now that you know why data security is important in healthcare, follow these five steps to start using it:
- Identify sensitive data.
Begin by identifying your most sensitive data by scanning all available resources and implementing appropriate security measures to protect it. When you know what data is on your servers and equipment, you can better protect it with cutting-edge techniques. This could mean using data masking or encryption to safeguard sensitive information.
- Do your research.
It’s important to stay informed about the latest security threats and trends by regularly reviewing industry news and participating in relevant training and conferences. This can help you stay ahead of potential vulnerabilities and emerging threats. By proactively staying in the know, you will maximize your data protection.
- Don’t Trust. Verify.
Implement strong access controls and permissions for your data to ensure that only authorized users can view or modify it. This can involve setting up multi-factor authentication and limiting user access based on job function or seniority. Because zero-trust architecture is necessary to fully protect all business systems and data, it’s now standard across all government systems.
- Continuously audit your systems.
Implementing security and data privacy is not a one-and-done deal. It requires constant vigilance. Regularly audit your systems and data to identify and address any vulnerabilities or weaknesses in your security protocols. This includes conducting security assessments, penetration testing, and vulnerability scans to identify and remediate security risks.
- Find the right solution.
When implementing data masking, it’s important to carefully evaluate and select a solution that meets your organization’s needs, budget, and compliance requirements. Consider factors such as ease of use, scalability, and data integrity when choosing a data masking tool. If the solution isn’t personalized to your organization’s specific requirements, it could cause more problems than it resolves.
- Apply masking when copying data to lower environments
Dev and test teams that need access to production-like data should leverage masking to ensure that no sensitive data makes it into lower environments; this enables them to freely leverage that data without risking data breaches or compliance violations.
Data masking is an important technique that protects sensitive data in various applications. It uses fake data to conceal real data from unauthorized users. When facing modern cyber threats, data masking is foundational to protecting your business data.