Table of Contents Hide
Is Data Objective? Unpacking the Reality
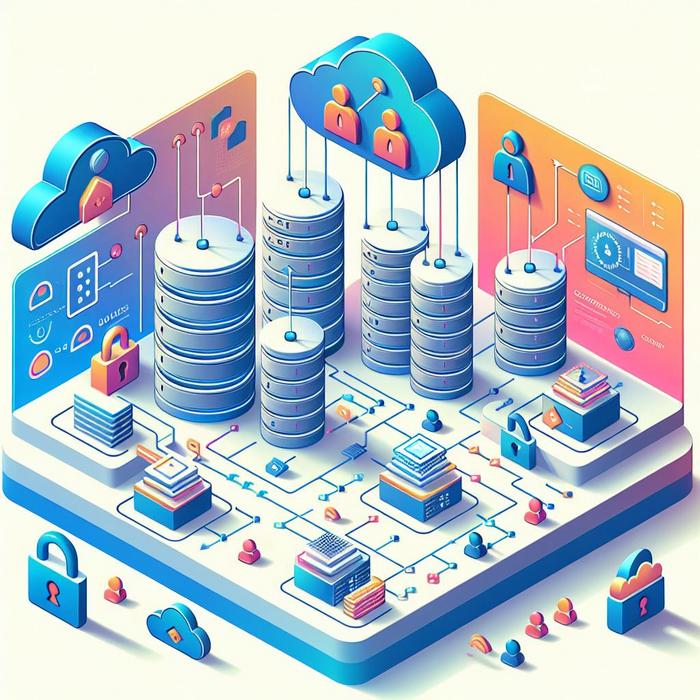
Does Zero Bias Exist in Data? Dissecting the Idea
How often does one examine and question the absolute objectivity of their data? Are we sure that our datasets are standing on solid foundations of impartiality? As professionals is managing data, we know too well that data is often believed to be objective. However, delving deeper into the intricacies of data handling, one learns to question this perceived absoluteness.
Unraveling the Objectivity Misapprehension
Data, much like information, is rendered meaningful in the context of its acquisition, application, and analysis. It is, therefore, critical to consider these aspects while questioning the objectivity of our datasets. While data anonymization techniques have leapfrogged in recent years, they have merely scratched the surface of ensuring complete objectivity and fairness.
Database virtualization is a revolutionary technique that has redefined the way data is accessed and utilized. By creating an abstract layer that provides unified access to data across different sources, this technology has fast-tracked data provisioning for various tasks, such as analytics and development. It has not only streamlined operations but has also ensured data reliability and reduced the odds of bias creeping into datasets.
But does the implementation of advanced solutions like database virtualization guarantee absolute objectivity in data? The answer is not black and white. Even with the best-in-class technologies and processes in place, the human factor remains a crucial variable affecting data objectivity.
Human Bias: The Oft-Overlooked Factor
Contrary to what some might believe, data does not exist in a vacuum. It is generated, collected, analyzed, and interpreted by individuals or systems built by humans, making it susceptible to preconceived notions and biases. As this article suggests, even supposedly ‘neutral’ algorithmic models can reflect and perpetuate biases.
An integral part of addressing this issue lies in being aware of this potential bias and its impact. The first step towards mitigating human bias is to acknowledge it, then devise strategies to restrict its influence on data. Some of these strategies might include ensuring diverse representation in every stage of data collection and interpretation, and regular audits of datasets and models.
Corporations and Objectivity
Within corporate contexts, especially those dealing with large datasets or complex data architectures, ensuring data objectivity poses a unique set of challenges. The role of executives such as CIOs, CTOs, data architects, and database administrators is pivotal in shaping strategies that are not only effective but also fair.
Notably, such leaders ought to consider how database virtualization impacts the objectivity within their organizations. Does flipping the switch to modern solutions like database virtualization automatically ensure bias-free data? Not necessarily. However, these technologies can significantly decouple data, rendering it less influenced by subjective factors, and making it more accessible and useful.
Agile strategies can be incorporated to bridge the gap between desired results and reality. By implementing these tactics, IT leaders can ensure that their information structures are not only functional but also embody the principles of fairness and neutrality.
Where Do We Go from Here?
While the path to complete objectivity in data might seem long and blurred, there is immense value in pursuing this goal. Recognizing the nuances, understanding the challenges, and adopting technologies like database virtualization can significantly contribute towards making data more objective.
The quest for zero-bias in data is a continuous process. Like any other journey, it starts with understanding the premise, evaluating the current scenarios, and then making informed decisions. Thus, as data architects and leaders, it’s essential not just to ask, “Is data objective,” but also to ponder, “How can we make our data more objective?”
These deliberations will not only lead to more efficient operations but also form the blueprint for a future where data can be truly equitable, reliable, and objective. As we tread on this path, it’s vital to realize that the journey towards data objectivity isn’t a destination, but an ongoing expedition full of exciting possibilities and insights. Is data objective? Perhaps, the more appropriate question to ask would be, can we make data more objective?
Navigating the Complexities of Data Anonymization
A key consideration in the pursuit of data objectivity is the practice of data anonymization. This critical process ensures data privacy by transforming identifiable data into unidentifiable information, thereby driving conclusions and decision-making based on objective information alone. However, data anonymization alone cannot guarantee complete objectivity. Therefore, organizations should combine database virtualization and data anonymization with other innovative methods to mitigate bias and ensure data objectivity.
Building on their vast experience, executives and data professionals should weigh the pros and cons of data anonymization and make informed decisions based on the specific needs of their business. Adopting a comprehensive approach that includes robust privacy regulations, ethical handling of data, and rigorous audits will contribute significantly to achieving data objectivity.
Embracing Innovative Solutions
Database virtualization has ushered in a new era of data operation optimization. As a powerful tool, it has made a significant impact in eliminating the barriers between various data sources, providing a more organized, easily manageable, and accessible data framework. Additionally, using advanced database virtualization techniques helps streamline operations, bolster data reliability, reduce data management costs, and importantly, mitigate bias creeping into datasets.
However, care should be taken when implementing these techniques. As these technologies become more sophisticated, data professionals and executives need to ensure that the principles of fairness and neutrality are not compromised. Adoption of agile methodologies, together with these advanced techniques, can ensure data integrity and reduce any form of biases.
Encouraging Diversity in Data Representation
One effective way to tackle potential human bias is by ensuring diverse representation in every stage of data collection and interpretation. By involving various perspectives, a broader analysis and interpretation of data are possible, potentially reducing the risks of unconscious bias affecting data objectivity. It’s crucial that companies remain vigilant to biases, constantly re-evaluating their approaches to gathering, interpreting, and acting upon the data they utilize.
A diverse team involved in data handling can critically evaluate the more subjective elements of data and minimize their influence on overall data interpretation.
Moving Forward: The Future is in Our Hands
The journey towards zero bias in data is continuous, evolving, and requires constant effort. While adopting advanced technologies like database virtualization and ongoing practices like data anonymization can make significant strides towards data objectivity, the role of human awareness and intervention remains pivotal.
Continuous learning, innovation, and flexibility are essential. As businesses grow and adapt to the ever-changing technological environment, it’s crucial to keep re-evaluating and refining data approaches to maintain the highest level of objectivity. Staying agile and being ready to pivot when necessary can make the difference between being simply data-driven and achieving true data objectivity.
A balanced and well-planned approach can mitigate risks and align data handling practices with the businesses’ overall objectives. By combining rigorous standards, regular audits, diversity in data handling, and advanced technologies, businesses can be on their way towards achieving data objectivity.
In conclusion, the question isn’t whether data can be truly objective; rather, it’s about the extents we can go as businesses and professionals to maximize data’s objectivity – thereby optimizing operations, driving innovation, and improving the quality of decision-making for a brighter, more prosperous future.
In this journey towards achieving data objectivity, the question remains – “What more can we do to make our data more objective?”.