Table of Contents Hide
The Potential Impact of RAG AI on Test Data Management
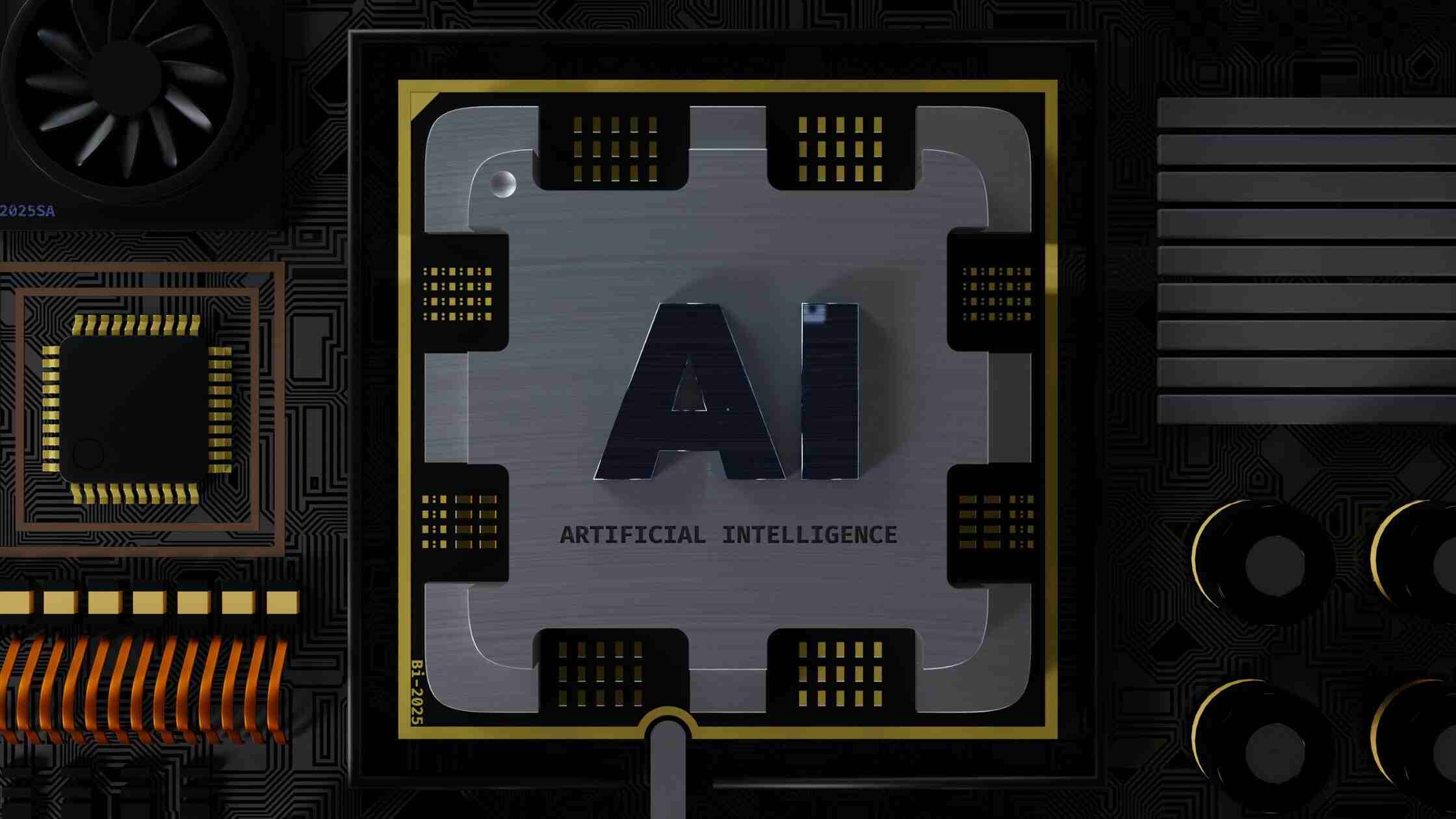
RAG AI and Test Data Management
In the rapidly evolving world of artificial intelligence (AI), innovations that blend the best of multiple approaches are gaining momentum. One such breakthrough is Retrieval Augmented Generation (RAG AI), an advanced AI technique combining information retrieval with generative AI. This hybrid model allows AI systems to retrieve relevant data from large datasets and use it to generate responses that are not only more accurate but also contextually relevant. This ability to enhance the output by leveraging external knowledge has positioned RAG AI as a key player in industries that rely heavily on data, including test data management (TDM).
The ability of RAG AI to process massive datasets and generate detailed outputs can provide significant benefits in scenarios where precision and timeliness are critical. In industries where quality testing is paramount, the intersection of RAG AI and TDM promises to revolutionize the way organizations manage and utilize test data. Let’s explore how this cutting-edge AI technology is poised to reshape the landscape of test data management, along with its technical challenges and broader implications for the future.
What is RAG AI?
At its core, RAG AI represents the fusion of two powerful AI paradigms: information retrieval and generative models. Traditional generative AI models, such as GPT, produce responses based solely on their internalized knowledge from training datasets. In contrast, Retrieval Augmented Generation AI goes a step further by retrieving external data and then generating responses using that retrieved information. This additional step allows the model to access more relevant, up-to-date, and accurate data, thereby improving the reliability and relevance of its outputs.
This approach enhances the overall performance of AI models by narrowing the gap between what AI can generate from its memory and what it can produce when armed with real-time information. In scenarios like test data generation or software testing environments, this precision is critical. Retrieval Augmented Generation AI ensures that the generated data is not only accurate but also reflective of actual, real-world scenarios, making it especially valuable in industries that require high fidelity and reliability.
How RAG AI Works
The core process behind RAG AI involves two primary steps: retrieval and generation.
- Retrieval: The AI first accesses a relevant knowledge base, which could be a database, external document, or dataset, depending on the query or task. For instance, if an organization uses RAG AI in the context of test data management, the system could access a database containing historical testing data, production data, or domain-specific knowledge.
- Generation: Once the information is retrieved, the generative AI uses this data to create context-specific responses. This is done by integrating the retrieved data with the model’s pre-existing knowledge, producing responses that are not only contextually accurate but also unique to the input query.
The result is a more dynamic, adaptable, and reliable system that can meet complex challenges—like generating varied and accurate test data—more effectively than traditional generative models.
Potential Impact of RAG AI on Test Data Management
Test data management has long been a critical aspect of software development, ensuring that systems are rigorously tested before they reach production. However, one of the ongoing challenges in TDM is the generation of diverse, relevant, and realistic test data that accurately mirrors production environments. This is where RAG AI could revolutionize the field.
Generating Realistic Test Data
The quality of a test largely depends on the quality of the test data used. In many cases, it can be difficult to replicate production data during the testing phase. However, by leveraging Retrieval Augmented Generation AI, organizations could generate synthetic test data that closely mimics real-world scenarios. This is done by retrieving actual production data and then using that data to generate synthetic counterparts that reflect the structure, variability, and nuances of real environments.
This capability is transformative because it not only enhances the accuracy of the testing process but also helps ensure that tests reflect the full range of possible scenarios the system will encounter post-launch. Teams could significantly improve the quality of their testing processes, resulting in fewer bugs and smoother software performance after release.
Enhancing Data Anonymization and Compliance
One of the biggest hurdles in modern test data management is ensuring compliance with data privacy laws such as GDPR and CCPA, which place strict requirements on how personal and sensitive data is handled. RAG AI could assist in data anonymization efforts by generating anonymized versions of datasets that retain essential characteristics for testing while safeguarding sensitive information.
For example, Retrieval Augmented Generation AI could retrieve real production data, anonymize sensitive fields, and generate a new test dataset that mirrors the original one. The result is a highly representative test dataset that meets compliance standards without compromising the integrity of the testing process. By making anonymization more effective, RAG AI helps organizations balance security with operational efficiency.
Technical Challenges in Implementation
Despite its numerous advantages, the integration of RAG AI into existing test data management systems is not without its challenges. Technical complexity and data quality are two primary areas where organizations may encounter obstacles.
System Complexity and Customization
Most test data management systems are highly customized to meet specific organizational needs. Integrating Retrieval Augmented Generation AI into these systems requires careful consideration and planning. Organizations must ensure that the AI-driven processes align with their existing workflows, data models, and standards. This often requires close collaboration between data scientists, AI engineers, and test management teams to ensure that the retrieval and generation of data are in sync with the company’s established practices.
Data Quality and Accessibility
The effectiveness of Retrieval Augmented Generation AI largely depends on the quality and structure of the data it can access. If the underlying datasets are poorly maintained or inconsistent, the output generated by RAG AI will suffer as well. This makes data curation and maintenance critical to successful implementation. Organizations need to invest in data cleaning and structuring efforts to ensure their datasets are comprehensive, well-organized, and accessible for AI retrieval.
While this process can be resource-intensive, the potential benefits in terms of testing accuracy and efficiency make it a worthwhile investment for companies that want to harness the full power of RAG AI in their test data management systems.
Broader Implications for the Future
The integration of RAG AI into test data management systems is just the beginning. As this technology continues to evolve, its potential applications could extend far beyond generating test data.
Automated, Intelligent Testing Environments
One of the most exciting possibilities is the creation of automated, intelligent testing environments. In such environments, RAG AI could continuously learn from previous testing cycles, dynamically adjusting the test data to reflect new requirements or address emerging issues. This would significantly reduce the amount of time and effort required for manual test data preparation, enabling teams to focus on more strategic, high-value tasks.
Advanced Simulations and Predictive Testing
As AI models become more sophisticated, Retrieval Augmented Generation AI could also enable advanced simulations and predictive testing. This would allow organizations to proactively identify and address potential system vulnerabilities before they become problems. The use of predictive analytics in testing could save valuable time and resources, ensuring that software products are not only functional but also resilient and future-proof.
Conclusion
RAG AI represents a paradigm shift in how organizations approach test data management. By combining the strengths of retrieval and generation, it offers unprecedented accuracy, efficiency, and data security. Although technical challenges remain, the potential benefits of integrating RAG AI into TDM systems are immense.
From generating more realistic test data to enhancing compliance and privacy, Retrieval Augmented Generation AI has the potential to transform testing processes in ways that were previously unimaginable. As industries continue to embrace AI-driven solutions, RAG AI could soon become a cornerstone of intelligent, automated, and predictive test data management systems, helping teams work smarter in an increasingly complex digital landscape.