Table of Contents Hide
Realistic Synthetic Data: Enhancing Test Data for Compliance & Software Development
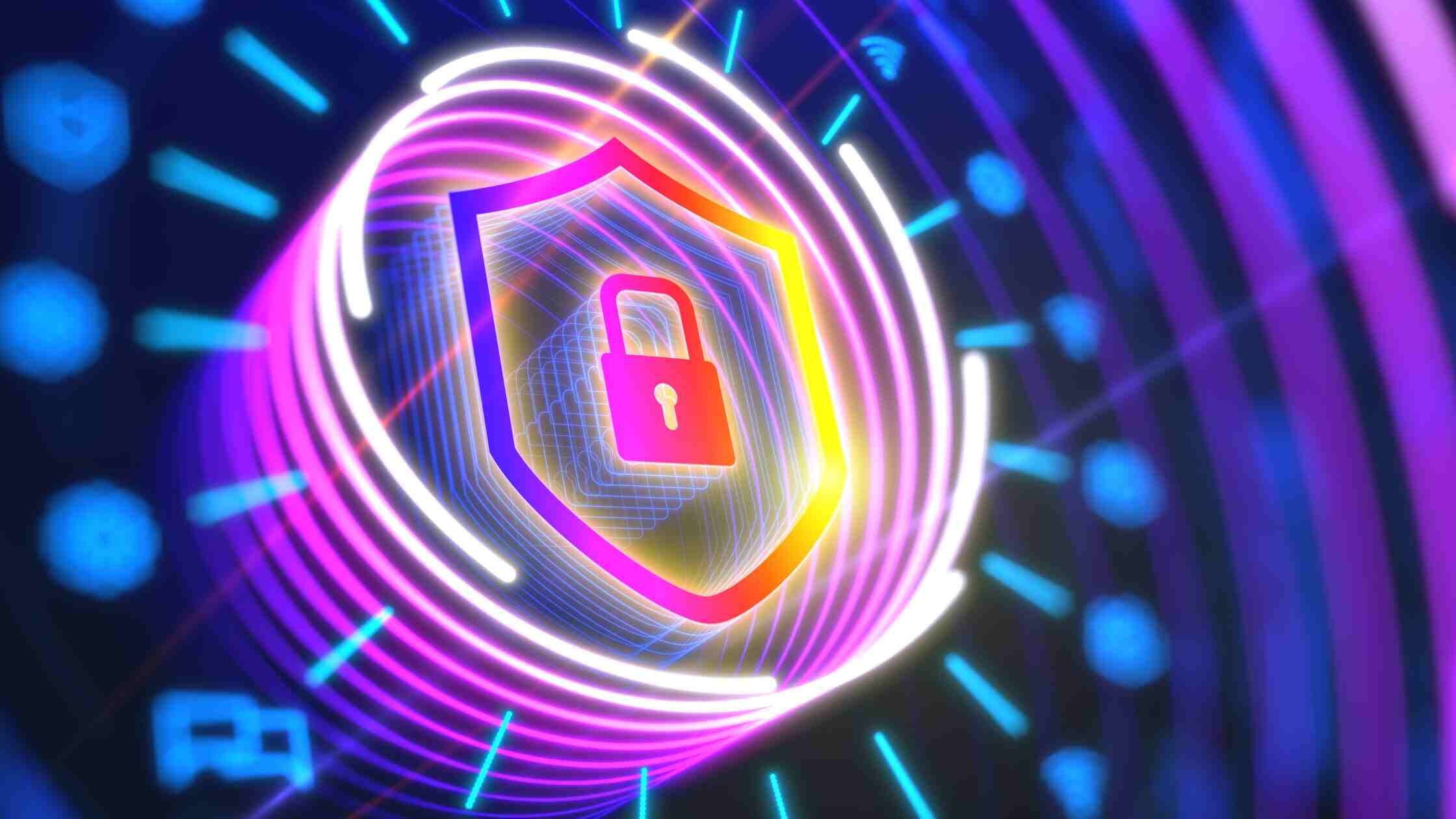
Realistic Synthetic Data: Transforming Data Privacy and Test Management
In an era where data privacy and regulatory compliance have become paramount, the concept of realistic synthetic data is emerging as a powerful tool for organizations. Test environments often require sensitive data that must be protected, but using real data can lead to compliance risks. This type of test data offers a groundbreaking solution by providing artificial datasets that resemble actual data closely while maintaining privacy and security. In this blog, we will explore what realistic synthetic data is, how it compares to traditional synthetic data, its role in compliance, and how Accelario’s data anonymization solution leverages AI to create realistic test data for various industries.
What is Realistic Synthetic Data?
Realistic synthetic data refers to artificially generated data that mimics the structure, behavior, and patterns of real-world data. Unlike purely synthetic data, which can sometimes lack the complexity or nuances of genuine datasets, realistic synthetic data provides a more accurate representation. This allows businesses to perform extensive testing and analytics without compromising sensitive information.
The critical difference between synthetic data and realistic synthetic data lies in the accuracy and usability of the latter. This type of test data is generated through advanced algorithms and AI technologies that replicate real-world data distributions, correlations, and trends. It’s not just random noise; it has meaning and context, making it more useful for testing, machine learning models, and simulations.
What is Synthetic Data?
Synthetic data is artificially created data that imitates real data sets. While traditional synthetic data serves its purpose in non-critical testing environments, it often lacks the depth and correlation found in real datasets. This limitation can lead to less reliable testing results and can miss certain edge cases that would only appear with real-world data patterns.
In contrast, realistic synthetic data provides a near-identical experience to real data, enabling organizations to conduct more precise tests and simulations. Whether for financial models, healthcare analytics, or software development, this type of data is indispensable for enhancing test accuracy without exposing sensitive information.
Realistic Synthetic Data vs Synthetic Data
While both synthetic data and realistic synthetic data are created artificially, there are some important differences:
- Accuracy: Traditional synthetic data is often simplified and lacks the complexity of real-world data. In contrast, realistic synthetic data closely replicates real data’s statistical distribution, making it far more accurate and applicable for real-world testing.
- Applicability: Because realistic synthetic data is more accurate, it is better suited for complex applications like software testing, fraud detection, and AI training. Regular synthetic data may fall short in these areas due to its lack of detail.
- Compliance: Both types of data protect privacy, but realistic synthetic data goes a step further by ensuring that the artificial data is indistinguishable from the real data, making it more valuable for industries that require high levels of data fidelity without compromising privacy regulations.
The Benefits of Realistic Synthetic Data
Here are a few compelling benefits of using realistic synthetic data in modern business environments:
- Data Privacy: Since realistic synthetic data contains no actual personal data, companies can reduce the risk of violating privacy regulations, such as GDPR or HIPAA.
- Cost Savings: Creating this type of test data reduces the need for accessing costly, restricted datasets. Instead, businesses can generate data tailored to their testing needs.
- Improved Testing Accuracy: Because it closely mimics real-world data, realistic test data provides more accurate testing environments for applications and systems, helping teams detect bugs, vulnerabilities, and performance bottlenecks more efficiently.
- Scalability: Traditional methods of acquiring data for testing purposes can be expensive and time-consuming. This type of test data can be generated in large quantities to meet the needs of growing organizations, all while maintaining data accuracy.
Realistic Synthetic Data and Compliance
One of the primary reasons businesses turn to realistic synthetic data is to ensure compliance with privacy regulations. With stringent data protection laws such as the General Data Protection Regulation (GDPR) and the California Consumer Privacy Act (CCPA), companies must be cautious when using personal data for testing or analysis.
This type of test data helps mitigate these risks by ensuring that the data used is not tied to any actual individuals, thus reducing the risk of data breaches. Since this synthetic data retains the characteristics of the original data, companies can confidently use it for tasks such as testing new software, analyzing trends, or training AI models, all while remaining compliant with data protection laws.
Realistic Test Data and Privacy
Testing applications with real data can often lead to breaches of privacy, especially in industries where data is highly sensitive, like finance or healthcare. By using realistic test data, organizations can simulate real-world testing conditions without compromising the privacy of actual users. This ensures that developers and testers can validate software behavior, check for bugs, and optimize performance using data that mirrors actual conditions, without risking compliance or violating privacy standards.
The Importance of Realistic Test Data
Accurate and realistic test data is essential for ensuring that systems, applications, and algorithms perform as expected under various scenarios. When test data is too simplistic, or unrelated to actual user behavior, the insights and results can be misleading. For example, testing a payment system with basic, unrealistic synthetic data could result in errors being missed or systems failing under real-world conditions.
By utilizing realistic synthetic data, businesses can emulate real-world scenarios, which is particularly useful for stress testing systems in industries like banking, healthcare, and telecommunications. Companies must ensure that the data used for testing reflects actual customer behavior, which is where realistic synthetic data steps in. This type of data allows developers to test systems with high levels of accuracy and variability while still protecting real data from exposure.
Accelario’s Data Anonymization and Realistic Test Data
Accelario’s Data Anonymization solution addresses one of the most pressing needs in today’s data-driven world—ensuring the security and privacy of sensitive information. Accelario’s AI-driven test data management solution creates realistic test data through advanced data anonymization techniques, ensuring that the data mirrors the complexities of real data while removing any identifiable information.
Accelario leverages AI to automatically detect patterns in data and generate synthetic counterparts that maintain statistical relevance. By doing so, organizations can perform development, testing, and QA work without ever exposing real data. This approach is not only highly secure but also fully compliant with global privacy regulations.
Key Benefits of Accelario’s Realistic Test Data:
- Enhanced Privacy: The data anonymization process ensures that no sensitive data is ever exposed, even in complex testing environments.
- Regulatory Compliance: With privacy regulations such as GDPR, HIPAA, and CCPA in place, realistic test data generated by Accelario ensures that companies remain compliant.
- AI-Driven Generation: By utilizing machine learning and AI algorithms, Accelario creates realistic test data that mirrors actual datasets, providing companies with highly accurate testing environments.
- Faster Time-to-Market: Realistic test data allows organizations to quickly deploy testing without the wait time associated with obtaining and anonymizing real data.
Why Businesses Should Adopt Using Realistic Test Data Now
The increasing demand for data-driven insights, combined with the growing complexity of regulatory landscapes, makes it essential for businesses to adopt it. Here are several key reasons why:
- Enhanced Security: Realistic synthetic data minimizes the risk of data breaches by eliminating the need to use real sensitive data during testing and development.
- Better Test Accuracy: By using data that closely mimics real-world conditions, businesses can improve the accuracy and reliability of their testing processes.
- Faster Time-to-Market: This type of test data accelerates the development cycle, allowing businesses to deploy new applications and services more quickly while ensuring compliance.
- Cost Efficiency: The ability to generate large volumes of synthetic data without relying on real-world data sources reduces the costs associated with data management and compliance.
Conclusion
Realistic synthetic data is revolutionizing how businesses test systems, develop AI models, and ensure compliance with privacy regulations. By creating data that closely mimics real-world datasets, companies can achieve high levels of accuracy in testing environments without compromising sensitive information. With the help of Accelario’s AI-driven test data management solution, organizations can stay ahead of the curve, leveraging realistic test data to innovate faster, safer, and more efficiently.